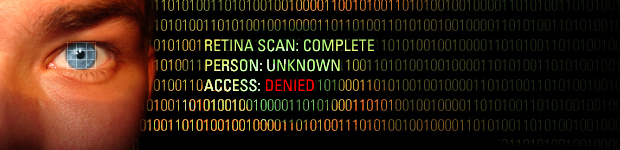
Australian Information Security Management Conference
Document Type
Article
Publisher
SRI Security Research Institute, Edith Cowan University, Perth, Western Australia
Abstract
Considering the significant volume of data generated by sensor systems and network hardware which is required to be analysed and intepreted by security analysts, the potential for human error is significant. This error can lead to consequent harm for some systems in the event of an adverse event not being detected. In this paper we compare two machine learning algorithms that can assist in supporting the security function effectively and present results that can be used to select the best algorithm for a specific domain. It is suggested that a naive Bayesian classiifer (NBC) and an artificial neural network (ANN) are most likely the best candidate algorithms for the proposed application. It was found that NBC was faster and more accurate than the ANN for the given data set. Future research will look to repeat this process for cyber security specific applications, and also examine teh GPGPU optimisations to the machine learning algorithms.
DOI
10.4225/75/57b65924343cd
Comments
11th Australian Information Security Management Conference, Edith Cowan University, Perth, Western Australia, 2nd-4th December, 2013