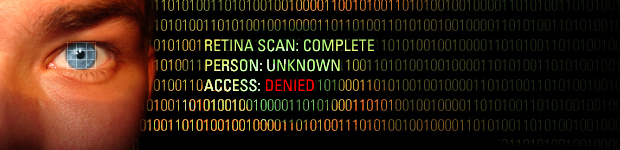
International Cyber Resilience conference
Document Type
Conference Proceeding
Publisher
School of Computer and Information Science, Security Research Centre, Edith Cowan University, Perth, Western Australia
Abstract
In this paper, we propose a five-step approach to detect obfuscated malware by investigating the structural and behavioural features of API calls. We have developed a fully automated system to disassemble and extract API call features effectively from executables. Using n-gram statistical analysis of binary content, we are able to classify if an executable file is malicious or benign. Our experimental results with a dataset of 242 malwares and 72 benign files have shown a promising accuracy of 96.5% for the unigram model. We also provide a preliminary analysis by our approach using support vector machine (SVM) and by varying n-values from 1 to 5, we have analysed the performance that include accuracy, false positives and false negatives. By applying SVM, we propose to train the classifier and derive an optimum n-gram model for detecting both known and unknown malware efficiently.
Comments
Originally published in the Proceedings of the 1st International Cyber Resilience Conference, Edith Cowan University, Perth Western Australia, 23rd August 2010